Next: Backpropagation through time
Up: Learning algorithms for DTRNN
Previous: Learning algorithms for DTRNN
  Contents
  Index
Gradient-based algorithms
The two most common gradient-based algorithms for DTRNN are backpropagation through time (BPTT) and real-time recurrent
learning (RTRL). Most other gradient-based algorithms may be
classified as using an intermediate or hybrid strategy combining the
desirable features of these two canonical algorithms.
The simplest
kind of gradient-based algorithm --used also for feedforward neural
networks-- is a
gradient-descent learning algorithm which updates
each learnable parameter
of the network according to the rule
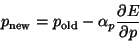 |
(4.26) |
where
is a positive magnitude (not necessarily a constant)
called the learning rate for parameter
and
is either the
total error for the whole
learning set (as in batch learning) or the error
for the pattern just presented (as in pattern learning). Most
gradient-based algorithms are improvements of this simple scheme (for
details see e.g. (Haykin, 1998, 220,233ff);
(Hertz et al., 1991, 103ff,123ff,157)); all of them require the
calculation of derivatives of error with respect to all of the
learnable parameters. The derivatives for a DTRNN may be computed (or
approximated) in different ways, which leads to a variety of methods.
Subsections
Next: Backpropagation through time
Up: Learning algorithms for DTRNN
Previous: Learning algorithms for DTRNN
  Contents
  Index
Debian User
2002-01-21